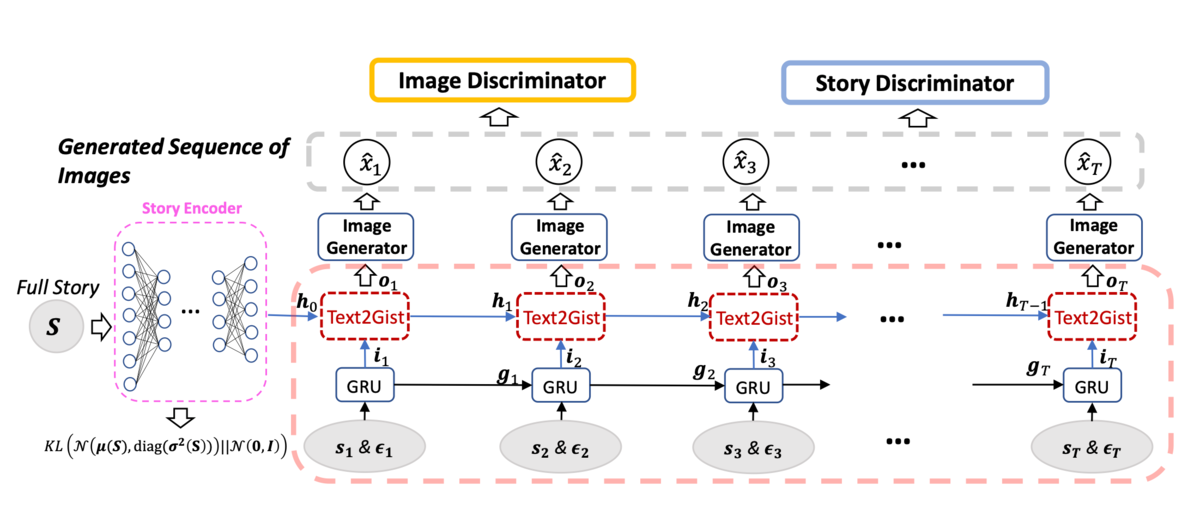
StoryGAN: A Sequential Conditional GAN for Story Visualization
openaccess.thecvf.comIn this work, we propose a new task called Story Vi- sualization. Given a multi-sentence paragraph, the story is visualized by generating a sequence of images, one for each sentence. In contrast to video generation, story vi- sualization focuses less on the continuity in generated im- ages (frames), but more on the global consistency across dy- namic scenes and characters – a challenge that has not been addressed by any single-image or video generation meth- ods. Therefore, we propose a new story-to-image-sequence generation model, StoryGAN, based on the sequential con- ditional GAN framework. Our model is unique in that it consists of a deep Context Encoder that dynamically tracks the story flow, and two discriminators at the story and im- age levels, to enhance the image quality and the consistency of the generated sequences. To evaluate the model, we mod- ified existing datasets to create the CLEVR-SV and Pororo- SV datasets. Empirically, StoryGAN outperformed state- of-the-art models in image quality, contextual consistency metrics, and human evaluation.